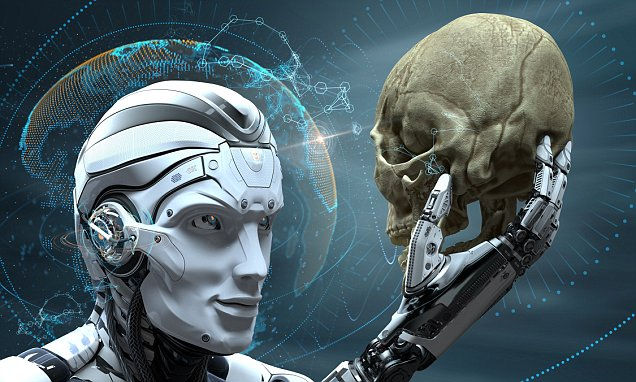
The history of artificial intelligence (AI), particularly artificial neural networks (ANNs), is a narrative characterized by successes, setbacks, and continuous innovation. From the inception of computational theories in the mid-20th century to the sophisticated architectures we have today, neural networks have evolved remarkably, encompassing diverse capabilities that have reshaped our interaction with technology.
The Birth of Neural Networks: 1940s to 1960s
The journey began in the 1940s with the pioneering works of neurophysiologist Warren McCulloch and mathematician Walter Pitts, who introduced a model of artificial neurons based on the functioning of biological neurons in 1943. Their work laid the theoretical foundation for ANNs, positing that neural networks could perform logical functions like the human brain. By the 1950s and early 1960s, the field gained attention thanks to figures like Frank Rosenblatt, whose 1958 invention of the Perceptron marked a significant advancement. The Perceptron was an early neural network capable of binary classification tasks. Despite its limitations, particularly its inability to handle non-linear separations, it inspired further research.
A perceptron is a single-layer neural network that takes one or more weighted inputs and outputs a single binary value, 0 or 1. Based on training data, a perceptron learns the weights of a decision boundary. It then iteratively adjusts the decision boundary until all samples are correctly classified. The perceptron's principles helped spark the modern artificial intelligence revolution.
The AI Winter: 1970s to 1980s
Interest in ANNs diminished during the 1970s, leading to what is commonly referred to as the "AI winter." The limitations of early neural networks, especially exposed by Marvin Minsky and Seymour Papert in their 1969 book, "Perceptrons," The AI winter is when interest in artificial intelligence (AI) research and development declined, resulting in reduced funding. Advances in the broader AI field stagnated, and the perceived over-promising of neural networks contributed to a decline in research funding.
Resurgence and Beyond: 1980s to 2000s
The revival of interest in ANNs surged in the mid-1980s due to several key breakthroughs. The introduction of backpropagation by Geoffrey Hinton, David Rumelhart, and Ronald J. Williams in 1986 enabled multilayer networks to learn from errors, reinvigorating interest in deeper architectures. Hinton, a central figure associated with the University of Toronto, and his collaborators contributed significantly to the capabilities of ANNs, demonstrating that they could learn complex patterns.
The late 1990s and early 2000s saw the emergence of various specialized neural network architectures. Convolutional Neural Networks (CNNs), popularized by Yann LeCun, excelled in image processing tasks, revolutionizing the field of computer vision. Recurrent Neural Networks (RNNs), capable of processing sequential data, found applications in natural language processing, with much of the foundational work attributed to researchers like Jürgen Schmidhuber.
Recent Developments and Innovations: 2010s to Present
The past decade has experienced breathtaking progress in ANN research, primarily driven by the development of robust computational hardware and vast amounts of data. Generative Adversarial Networks (GANs), introduced by Ian Goodfellow in 2014, highlighted a novel approach to data generation, utilizing two neural networks in competition to produce increasingly realistic outputs.
Long-short-term memory Networks (LSTMs), a specialized form of RNNs, have enabled significant advancements in tasks requiring long-term dependencies, such as speech recognition and language translation. Innovations in Radial Basis Function Networks (RBFNs) have exhibited faster learning speeds, broadening the applicability of ANNs.
The Future Landscape of Artificial Neural Networks
Looking toward the future, ANNs will likely continue to play an integral role in AI's evolution. Key stakeholders will drive further research and development, including academic institutions, tech giants, and government bodies. Universities like Stanford and MIT remain at the forefront, fostering innovation and interdisciplinary collaboration. Private companies, such as Google and OpenAI, invest heavily in advancing neural architectures, pushing the boundaries of what is possible with AI.
As ANNs become more sophisticated, ethical considerations will be essential in their deployment. Responsible AI initiatives will be crucial in ensuring that advances in neural networks do not lead to unintended consequences. Integrating AI into sectors such as healthcare, finance, and autonomous systems will necessitate ongoing dialogue among researchers, policymakers, and industry leaders.
Conclusion
The history of artificial neural networks is a testament to human ingenuity and resilience. From humble beginnings, the field has witnessed cycles of optimism and despair, leading to a renaissance in AI capabilities. Moving forward, the continued collaboration among key stakeholders will be paramount as we navigate the complex landscape of neural networks, ensuring that their powerful potential is harnessed for the betterment of society.
Comments