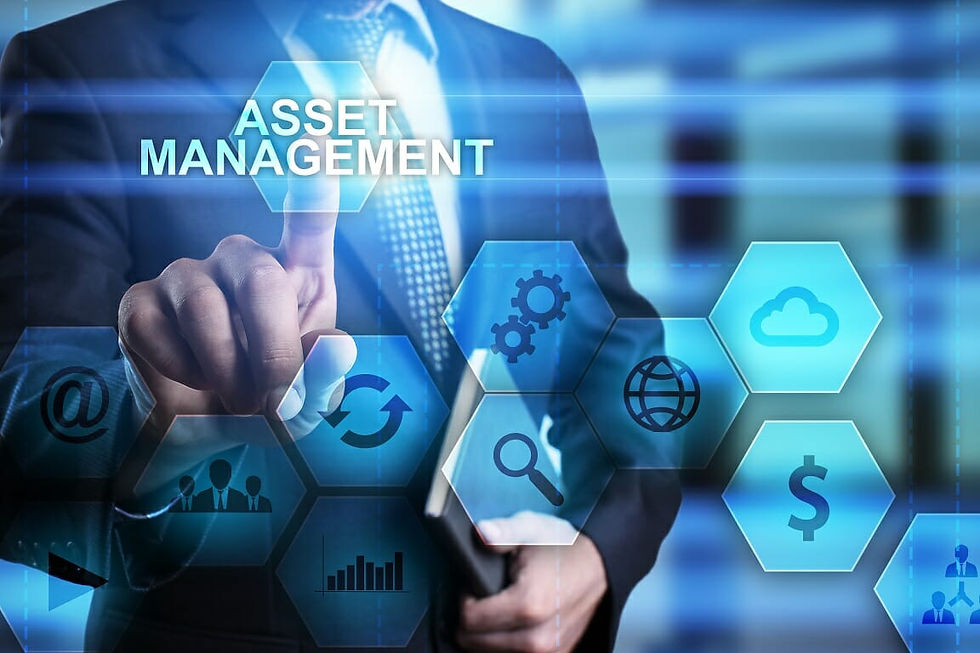
Machine Learning can potentially reverse the increasing trend toward passive investment funds in the asset management industry. With its ability to analyze large data sets and generate predictions, Machine Learning offers new tools that could help active investors outperform the indexes. However, whether this technology will deliver a sustainable business model for active asset managers is still being determined.
One of the critical advantages of Machine Learning in securities investing is its adaptability and efficiency. Unlike other sectors where regulatory approvals are required, portfolio managers can translate Machine Learning insights into investment decisions without any hindrances. Machine Learning can identify potentially outperforming equities by finding new patterns in existing data sets and generate insights applicable to current CEOs' statements by analyzing historical data. It can also improve accuracy in predicting bond defaults by around 10% compared to traditional models.
Another significant advantage of Machine Learning is its ability to make new forms of data analyzable. Alternative datasets like GPS locations from mobile phones or point-of-sale data can be used to predict company performance. By examining vast amounts of data in various formats, Machine Learning algorithms can detect useful trends to help investment decision-making.
Machine Learning also helps reduce the adverse effects of human biases on investment decisions. By analyzing historical trading records, Machine Learning algorithms can identify patterns manifesting bias, enabling individuals to double-check and mitigate unhelpful patterns at every level of the investment process.
However, Machine Learning has its limitations. Biases derived from data sources or algorithm deficiencies can affect the accuracy of predictions. Machine Learning also struggles with rare or unprecedented situations that do not correlate clearly to past trends. Additionally, many patterns identified by Machine Learning are just correlations and do not reveal the underlying drivers, requiring skilled professionals to differentiate between signal and noise.
Asset management firms must invest in technology and skilled personnel to fully utilize Machine Learning. Despite its potential, most asset managers still rely on traditional tools like Excel spreadsheets. This slow adoption can be attributed to the need for more technological infrastructure, costs associated with implementation, and the downward pressure on fees in the industry.
While Machine Learning can significantly improve data analysis quality, it cannot replace human judgment. Asset management firms must balance technology's capabilities and human expertise. While larger firms are leading the way in adopting Machine Learning, mid-size firms can also benefit due to their ability to attract and retain data scientists.
Ultimately, it remains to be seen whether Machine Learning investment strategies can create a sustainable business model for active asset managers. The uniqueness of alpha generated by Machine Learning may be short-lived as other managers catch up and simulate the same methods. This could lead to the simultaneous buying or selling of securities, potentially erasing any gains. Moreover, the industry's current shift towards passive investing and decreasing fees pose additional challenges for small asset management firms, making substantial investments in Machine Learning difficult to sustain.
In conclusion, Machine Learning holds promise for active investing by enabling early adopters to find new sources of alpha. However, replicating insights by other managers and the potential for simultaneous trading of securities may limit the long-term viability of Machine Learning as a sustainable business model for active asset managers. The industry must carefully navigate these challenges and balance technology and human judgment to ensure its success.
Comments