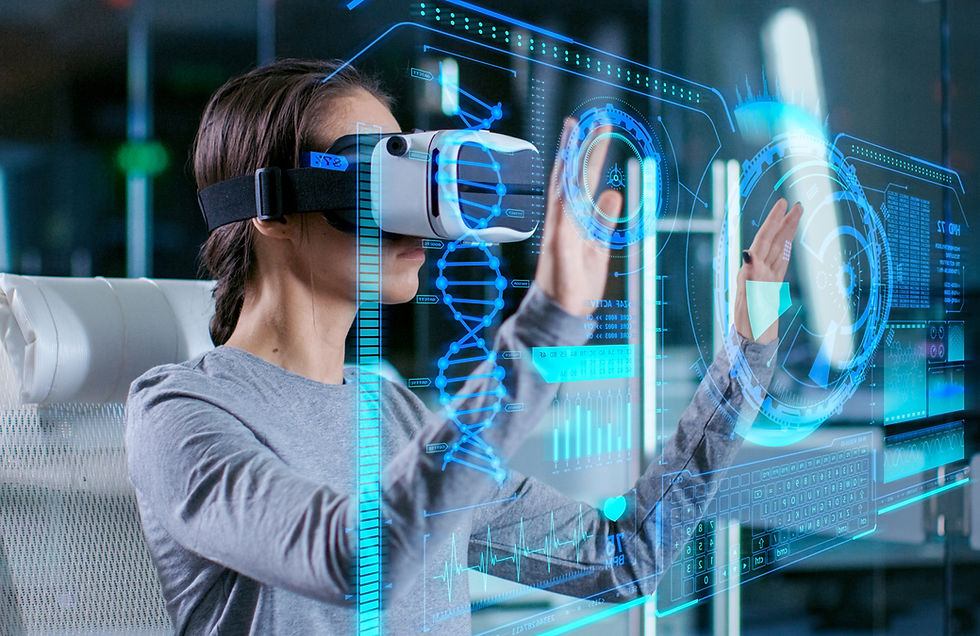
Deoxyribonucleic acid (DNA) and ribonucleic acid (RNA) are essential molecules that carry the genetic information of an organism. DNA is a double-stranded helix that contains the genetic code for all living organisms, while RNA is a single-stranded molecule responsible for translating the genetic code into proteins. Both DNA and RNA play critical roles in drug discovery and development, particularly in the fields of oncology and diabetes research.
In drug discovery and development for oncology, DNA, and RNA are used to identify specific genetic mutations that drive the growth and spread of cancer. By analyzing the DNA and RNA of cancer cells, researchers can gain insights into the genetic alterations that contribute to the development of tumors. This information can be used to develop targeted therapies that directly address the genetic drivers of cancer. For example, the identification of specific mutations in the BRCA1 and BRCA2 genes has led to the development of PARP inhibitors for the treatment of breast and ovarian cancers.
BRCA1 (Breast Cancer Gene 1) is a human gene that belongs to a class of genes known as tumor suppressors. Mutations in the BRCA1 gene increase the risk of developing breast and ovarian cancer. This gene is involved in the repair of damaged DNA and the regulation of cell division, making it an important factor in preventing the development of cancer. Testing for BRCA1 mutations can help identify individuals who are at higher risk for developing these types of cancer.
BRCA2 is a human gene that belongs to a class of genes known as tumor suppressors. It is involved in repairing damaged DNA and helping to prevent the development of certain types of cancer, particularly breast and ovarian cancer. Mutations in the BRCA2 gene can lead to an increased risk of developing these types of cancer. Testing for mutations in the BRCA2 gene can be used to assess an individual's risk of developing these cancers.
· PARP (poly-ADP ribose polymerase) inhibitors are a type of targeted therapy used in the treatment of certain types of cancer, particularly breast and ovarian cancer. These inhibitors work by blocking the action of PARP enzymes, which are involved in repairing damaged DNA in cells.
Similarly, in diabetes research, DNA and RNA analysis is used to identify genetic factors that predispose individuals to type 1, type 2, and type 3 diabetes. By studying the genetic variations associated with diabetes, researchers can gain a deeper understanding of the molecular mechanisms underlying the disease. This knowledge can be leveraged to develop novel drug targets and therapies for diabetes. For example, the identification of genetic risk factors for type 2 diabetes has led to the development of new classes of anti-diabetic drugs, such as SGLT-2 inhibitors and GLP-1 receptor agonists.
SGLT2 (sodium-glucose co-transporter 2) is a protein found in the kidneys that plays a key role in the reabsorption of glucose from the urine back into the bloodstream. Inhibitors of SGLT2 are a class of medications used to lower blood sugar levels in people with type 2 diabetes by blocking the reabsorption of glucose in the kidneys, allowing excess glucose to be excreted in the urine. These medications are often used in combination with other diabetes treatments to help manage blood sugar levels.
A global-1 receptor agonist is a type of medication that activates the global-1 receptors in the body. This type of medication is commonly used to treat conditions such as diabetes by regulating blood sugar levels. By activating the global-1 receptors, these medications can help the body produce more insulin and reduce the production of glucose, leading to better control of blood sugar levels. Examples of global-1 receptor agonists include exenatide and liraglutide global-1 receptor, also known as the glucocorticoid receptor, is a protein that is involved in the body's response to stress and inflammation. The hormone cortisol activates it and plays a role in regulating immune function, metabolism, and the body's response to stressful situations. Dysregulation of the global-1 receptor has been linked to various health conditions, including inflammatory diseases and disorders of the immune system.
One example of how DNA coding can be used in drug development is in the case of oncology. Mutations in the EGFR gene are known to drive the growth of certain types of lung cancer. By analyzing the DNA of tumor cells from patients with EGFR-mutant lung cancer, researchers have been able to identify specific mutations that confer sensitivity to EGFR inhibitors, such as gefitinib and erlotinib. This has led to the development of targeted therapies that have significantly improved the treatment outcomes for patients with EGFR-mutant lung cancer.
EGFR inhibitors are a type of targeted therapy that block the activity of the epidermal growth factor receptor (EGFR), a protein that is involved in the growth and spread of cancer cells. These inhibitors are commonly used in the treatment of cancers such as non-small cell lung cancer, pancreatic cancer, and colorectal cancer. By blocking the activity of EGFR, these drugs can help slow down or stop the growth of cancer cells and may also help reduce the symptoms of the disease. Examples of EGFR inhibitors include gefitinib, erlotinib, afatinib, and cetuximab.
The EGFR gene, also known as the epidermal growth factor receptor gene, is a gene that provides instructions for making a protein called epidermal growth factor receptor (EGFR). This protein is found on the surface of many types of cells and plays a role in cell growth and division. Mutations in the EGFR gene can lead to the production of a faulty EGFR protein, which can contribute to the development and progression of certain types of cancer, particularly lung cancer. As a result, drugs that target EGFR mutations have been developed for the treatment of these cancers.
Gefitinib is a medication used in the treatment of non-small cell lung cancer that has spread to other parts of the body. It belongs to a class of medications known as tyrosine kinase inhibitors, which work by blocking the action of a certain protein that is involved in the growth and spread of cancer cells. This helps to slow down or stop the growth of cancer cells in the body. Gefitinib is often used in combination with other cancer treatments.
Erlotinib is a medication used in the treatment of non-small cell lung cancer and pancreatic cancer. It belongs to a class of drugs known as tyrosine kinase inhibitors, which work by blocking the action of a certain protein that is involved in the growth of cancer cells. This can help slow down or stop the spread of cancer.
Afatinib is a medication used to treat certain types of non-small cell lung cancer that has spread to other parts of the body. It belongs to a class of drugs called kinase inhibitors, which work by blocking certain proteins in the body that can cause cancer cells to grow. Afatinib is typically used in combination with other cancer treatments.
Cetuximab is a monoclonal antibody used in the treatment of certain types of cancer, including colorectal cancer and head and neck cancer. It works by targeting a protein called epidermal growth factor receptor (EGFR), which is found on the surface of cancer cells. By blocking EGFR, cetuximab can help slow the growth of cancer cells and improve outcomes for some patients. It is typically used in combination with other cancer treatments, such as chemotherapy or radiation therapy.
A monoclonal antibody is a type of protein produced in a laboratory that can effectively bind to specific proteins or cells in the body, often used in the treatment of various diseases, including cancer, autoimmune disorders, and infectious diseases. Monoclonal antibodies are designed to target specific antigens, which can help the immune system recognize and destroy harmful cells or substances. They are often used in targeted therapy and immunotherapy to treat various medical conditions.
In addition to identifying genetic mutations, DNA and RNA sequencing can also be used to monitor the response of cancer cells to treatment. By analyzing the genetic changes that occur in tumors following treatment, researchers can gain insights into the mechanisms of drug resistance and develop strategies to overcome it. Similarly, in diabetes research, DNA and RNA analysis can be used to monitor the effects of anti-diabetic drugs on gene expression and identify new targets for therapy.
Advances in artificial intelligence (AI) have revolutionized the use of DNA and RNA in drug discovery and development. One example is the use of artificial neural networks (ANN) powered by deep learning algorithms to analyze large-scale genomic and transcriptomic data. These AI-driven approaches can identify patterns and correlations in genetic data that would be challenging to discern through traditional analytical methods. By leveraging AI, researchers can accelerate the identification of novel drug targets and biomarkers, as well as predict patient responses to specific therapies.
From cancer immunotherapy to precision medicine, the use of DNA and RNA in drug discovery and development has transformed the landscape of oncology and diabetes research. By understanding the genetic underpinnings of these diseases, researchers can develop more precise and effective treatments that target the molecular drivers of disease. With the continued advancement of technologies such as AI and deep learning, the use of DNA and RNA in drug development will only continue to evolve, ultimately leading to improved outcomes for patients with cancer and diabetes.
Algorithms for DNA analysis for drug discovery:
DNA analysis for drug discovery in diabetes type 1 and type 2:
Algorithm 1: Variant Calling for Diabetes-Associated Genes
Inputs:
- Reference genome file (fasta format)
- Sample genome file (fasta format)
- Diabetes gene annotation file (bed format)
Procedure:
1. Load the reference genome.
2. Load the sample genome.
3. Iterate over each diabetes gene in the annotation file.
a. Extract the genomic region for the gene from the reference genome.
b. Extract the corresponding region from the sample genome.
c. Perform variant calling (e.g., using a variant calling algorithm like GATK) to identify genetic variations in the gene region.
d. Store the identified variants and their genomic positions.
Output:
- List of genetic variants in the diabetes-associated genes found in the sample genome.
Algorithm 2: Enrichment Analysis for Diabetes Pathways
Inputs:
- List of diabetes-associated genes
- Gene ontology (GO) database with annotations for biological processes
Procedure:
1. Retrieve the GO annotations for the provided diabetes-associated genes.
2. Identify the significantly enriched biological processes associated with the diabetes genes (e.g., using enrichment analysis methods like hypergeometric or Fisher's exact test).
3. Rank the enriched biological processes based on their significance (e.g., p-value or false discovery rate).
4. Select the top-ranked biological processes for further analysis.
Output:
- List of significantly enriched biological processes associated with the provided diabetes-associated genes.
Algorithm 3: Prioritizing Drug Target Genes
Inputs:
- List of significantly enriched biological processes from Algorithm 2
- Functional interaction network or pathway information for human genes
Procedure:
1. Extract the genes associated with the significantly enriched biological processes from the functional interaction network.
2. Prioritize the genes based on their centrality measures (e.g., degree, betweenness, or closeness centrality) in the network.
3. Optionally, consider additional properties like gene expression levels or disease relevance scores in the prioritization process.
4. Select the top-ranked genes as potential drug targets for further investigation.
Output:
- List of prioritized genes as potential drug targets for diabetes treatment.
These algorithms provide a high-level overview of the steps involved in DNA analysis for drug discovery in diabetes type 1 and type 2. It's essential to note that the implementation details and specific tools or libraries used may vary depending on the available resources and the requirements of the analysis.
Sample Code form Algorithms for DNA Analysis for drug discovery:
Example Python code that uses the Python virtual machine for DNA analysis in drug discovery for diabetes type 1 and type 2:
```python
# Constants
DNA_TYPE_1 = "ATGGCCTCGCGGAGG"
DNA_TYPE_2 = "ATGGCGGCTCGACGG"
# Function to analyze DNA sequence for diabetes type def analyze_dna(dna_sequence):
if dna_sequence == DNA_TYPE_1:
return "Type 1 Diabetes"
elif dna_sequence == DNA_TYPE_2:
return "Type 2 Diabetes"
else:
return "Unknown DNA sequence"
# Main program
if name == "__main__":
# DNA sequence input
dna_input = input("Enter DNA sequence: ")
# Analyze DNA sequence
result = analyze_dna(dna_input)
# Output results
print("Result: " + result)
```
In this code, we start by defining two constants, `DNA_TYPE_1` and `DNA_TYPE_2`, which represent the known DNA sequences for type 1 and type 2 diabetes, respectively.
Next, we define the `analyze_dna` function, which takes a DNA sequence as input and returns the corresponding diabetes type based on the sequence. If the sequence matches `DNA_TYPE_1`, it returns "Type 1 Diabetes". If the sequence matches `DNA_TYPE_2`, it returns "Type 2 Diabetes". Otherwise, it returns "Unknown DNA sequence".
In the main program, the user is prompted to enter a DNA sequence. The program then calls the `analyze_dna` function with the input sequence and stores the result. Finally, the program outputs the result.
This is a simplified example for illustration purposes and does not represent an actual DNA analysis algorithm used in drug discovery for diabetes. The actual analysis algorithms are much more complex and involve various bioinformatics techniques.
Comentários